Keynote
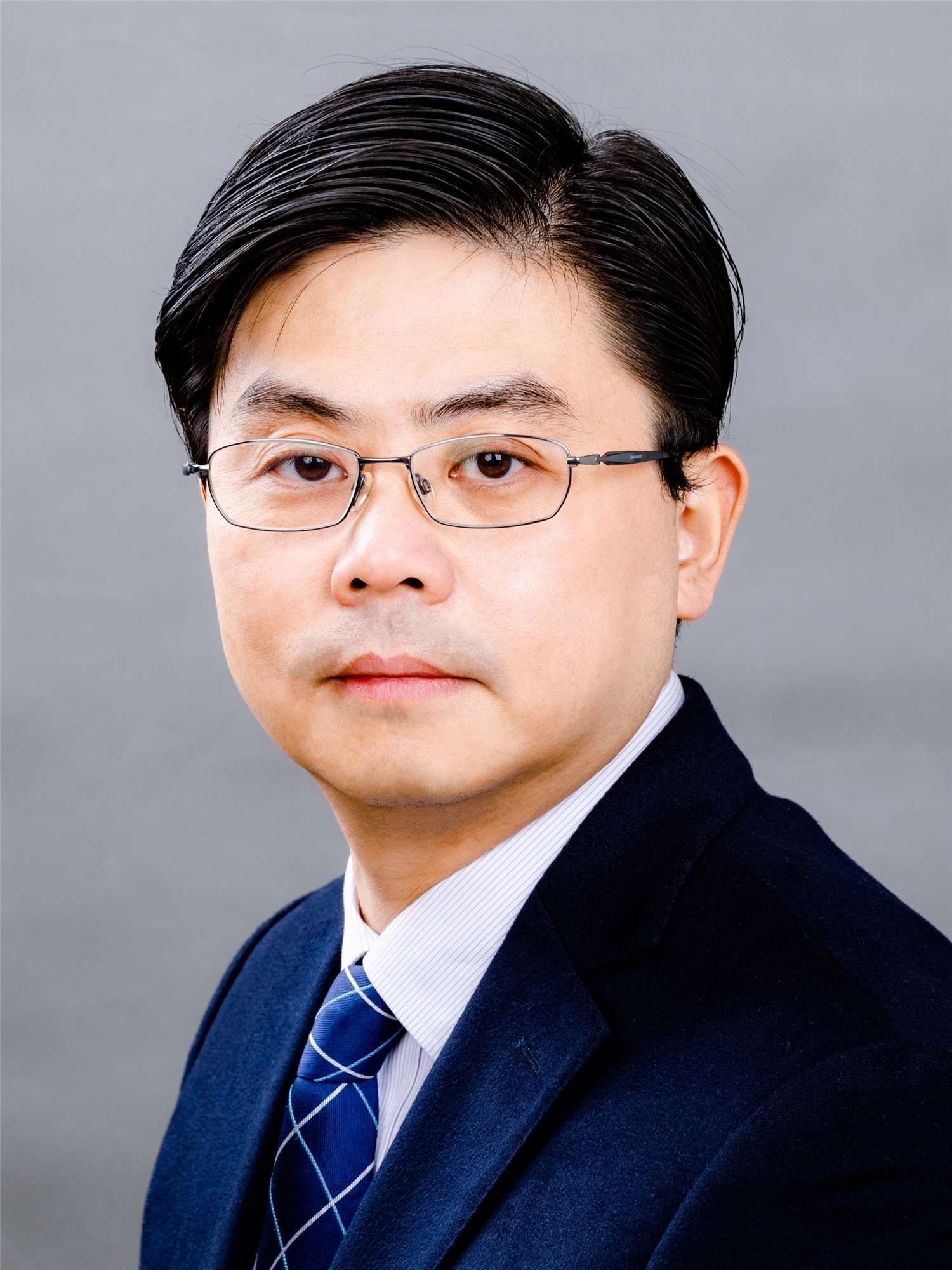
方方 教授
视觉学习与成人大脑的可塑性
第一部分,介绍实验室近10年来知觉学习脑成像领域研究进展和为未来发展方向的思考。
第二部分,介绍北京大学(尤其是心理与认知科学学院、北大-清华生命科学联合中心、麦戈文脑科学研究所、北京大学人工智能研究院)对青年人才的招聘和培养。
Neuroimaging
Ting Xu
The introduction of PRIME-DE
Nonhuman Primate (NHP) neuroimaging is a rapidly maturing area for advancing translational neuroscience and linking macroscale observations to underlying meso- and microscale phenomena. The recently established PRIMatE Data Exchange (PRIME-DE) - a NHP neuroimaging data consortium - have aggregated and shared 225 primates from 26 laboratories throughout the world, which provides unique opportunities to the NHP community. In this presentation, I will introduce PRIME-DE, the relevant NHP resources, challenges and potential solutions in data assessment and analytic pipeline, as well as the current progress in within- and across-species alignment.
Xin Di
Estimations of the weather effects on brain functions using functional MRI – a cautionary tale
The influences of environmental factors such as weather on the human brain are still largely unknown. A few neuroimaging studies have demonstrated seasonal effects, but were limited by their cross-sectional design or sample sizes. Most importantly, the stability of MRI scanners hasn’t been taken into account, which may also be affected by environments. In the current study, we analyzed longitudinal resting-state functional MRI (fMRI) data from eight individuals, where the participants were scanned over months to years. We applied machine learning regression to use different resting-state parameters, including amplitude of low-frequency fluctuations (ALFF), regional homogeneity (ReHo), and functional connectivity matrix, to predict different weather and environmental parameters. For a careful control, the raw EPI and the anatomical images were also used in the prediction analysis. We first found that daylight length and temperatures could be reliably predicted using cross-validation using resting-state parameters. However, similar prediction accuracies could also be achieved by using one frame of EPI image, and even higher accuracies could be achieved by using segmented or even the raw anatomical images. Finally, we verified that the signals outside of the brain in the anatomical images and signals in phantom scans could also achieve higher prediction accuracies, suggesting that the predictability may be due to the baseline signals of the MRI scanner. After all, we did not identify detectable influences of weather on brain functions other than the influences on the stability of MRI scanners. The results highlight the difficulty of studying long term effects on the brain using MRI.
Chao-Gan Yan
The REST-meta-MDD Project: towards a Neuroimaging Biomarker of Major Depressive Disorder
Major Depressive Disorder (MDD) is common and disabling, but its neuropathophysiology remains unclear. Most studies of functional brain networks in MDD have had limited statistical power and data analysis approaches have varied widely. The REST-meta-MDD Project of resting-state fMRI (R-fMRI) addresses these issues. Twenty-five research groups in China established the REST-meta-MDD Consortium by contributing R-fMRI data from 1,300 patients with MDD and 1,128 normal controls (NCs). Data were preprocessed locally with a standardized protocol prior to aggregated group analyses. We focused on functional connectivity (FC) within the default mode network (DMN), frequently reported to be increased in MDD. Instead, we found decreased DMN FC when we compared 848 patients with MDD to 794 NCs from 17 sites after data exclusion. We found FC reduction only in recurrent MDD, not in first-episode drug-naïve MDD. Decreased DMN FC was associated with medication usage but not with MDD duration. DMN FC was also positively related to symptom severity but only in recurrent MDD. Exploratory analyses also revealed alterations in FC of visual, sensory-motor and dorsal attention networks in MDD. We confirmed the key role of DMN in MDD but found reduced rather than increased FC within the DMN. All resting-state fMRI indices of data contributed by the REST-meta-MDD consortium are being shared publicly via the R-fMRI Maps Project. As a next step, the REST-meta-MDD consortium is going to collaborate with interactional MDD researchers, to build an international brain imaging big database of depression, investigate the ethnic-general and ethnic-specific brain pattern in depression, and use deep learning and transfer learning to build a deep neural network to classify MDD patients. This project will help shed new light on identifying biomarkers for depression's clinical diagnosis and treatment.

Hengyi Cao
Towards the understanding of state-independent neural traits underlying the pathogenesis of schizophrenia
While schizophrenia is associated with symptoms in multiple psychological dimensions, traditional fMRI research based on a single paradigm bears limited capability of identifying state-independent neural traits that account for various psychopathological aspects of this complex disorder. Here we present our recent work that leverages multi-paradigm fMRI data and a cross-paradigm connectivity method to study functional “trait” abnormalities that index the polygenic risk and potentially predict the future onset of schizophrenia. We start with the demonstration that the inclusion of multiple paradigms would significantly increase the reliability and individual identifiability of human functional connectome, when compared with single-paradigm data. Using cross-paradigm connectivity, we then further show that 1) schizophrenia polygenic risk is associated with trait-like connectivity decreases in the frontoparietal network, default-mode network, and visual network; and 2) increased connectivity in the cerebello-thalamo-cortical circuitry is a trait-like predictor for future onset of schizophrenia among individuals at high risk. Both neural traits are highly correlated, suggesting interrelated mechanisms that jointly contribute to the pathogenesis of schizophrenia. These findings highlight the utility of using multi-paradigm connectivity data to understand the fundamental mechanisms of schizophrenia and clinical disorders.
Quanying Liu
The networks and layered architectures of brain
In an idling condition, the brain is thought to prepare itself for future demands by generating coordinated dynamics that largely overlap with patterns of previous activity. These coordinated dynamics can be studied by using functional connectivity methods. I reported - for the first time – the successful brain network imaging using. Here, I will present some methodological approaches that are needed to improve the spatial resolution of source localization with high-density EEG (hdEEG), and to permit brain network imaging using this technique. In particular, we have developed tools for signal preprocessing, head modeling, neural activity reconstruction and connectivity analysis. The methods we developed for resting data analysis is applicable to the task-related data.
Mingbo Cai
Incorporating structured assumptions with probabilistic graphical models in fMRI data analysis
With the wide adoption of functional magnetic resonance imaging (fMRI) by cognitive neuroscience researchers, large volumes of brain imaging data have been accumulated in recent years. Aggregating these data to derive scientific insights often faces the challenge that fMRI data are high-dimensional, heterogeneous across people, and noisy. These challenges demand the development of computational tools that are tailored both for the neuroscience questions and for the properties of the data. We review a few recently developed algorithms in various domains of fMRI research: fMRI in naturalistic tasks, analyzing full-brain functional connectivity, pattern classification, inferring representational similarity and modeling structured residuals. These algorithms all tackle the challenges in fMRI similarly: they start by making clear statements of assumptions about neural data and existing domain knowledge, incorporating those assumptions and domain knowledge into probabilistic graphical models, and using those models to estimate properties of interest or latent structures in the data. Such approaches can avoid erroneous findings, reduce the impact of noise, better utilize known properties of the data, and better aggregate data across groups of subjects.With these successful cases, we advocate wider adoption of explicit model construction in cognitive neuroscience.
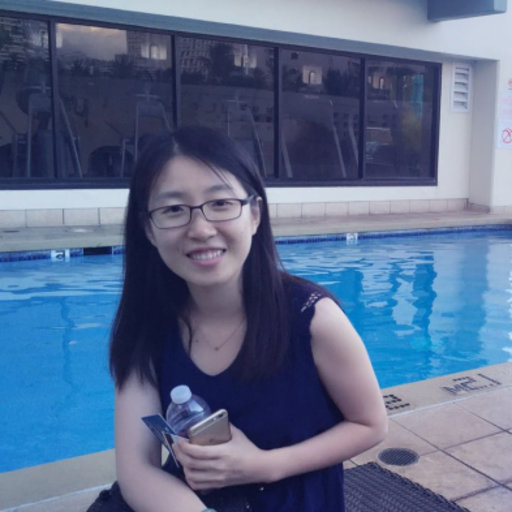
Miao Cao
Development of language network- evidence from fMRI
Language is the way and tool for human communication, which rapidly acquired with development. Human infants are born with a set of “equipment“ to acquire speech spontaneously when provided with enough language exposure. However, how these “equipment” develops before birth and the relationship between early development of them and later language performance remain largely unknown. We employed the resting-state fMRI data of preterm babies, term babies and adults to explore the development of auditory language processing networks during the 3rd trimester and how they influence the language performance at 2 years old. In addition to auditory language processing, visual language processing, especially reading, is an important skill for human beings to obtain information, whose acquisition become a major learning task for children. In another work, we explored the neural mechanism of reading skill acquisition in primary school students. We compared whole brain networks of children and adults based on their task-fMRI data during text reading to explore the regional, connectional and modular changes with development.
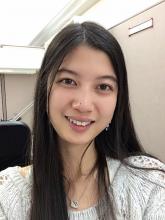
Jingyuan Chen
Resting-state "physiological networks”
Slow changes in systemic brain physiology can elicit large fluctuations in fMRI time series, which manifest as structured spatial patterns of temporal correlations between distant brain regions. In this talk, we show that such “physiological networks”—sets of segregated brain regions that exhibit similar responses following slow changes in systemic physiology—resemble patterns associated with large-scale networks typically attributed to remotely synchronized neuronal activity. We will further show that such physiologically-relevant connectivity estimates appear to dominate the overall connectivity observations in multiple datasets from the 3T Human Connectome Project, and that this apparent “physiological connectivity” cannot be removed by the use of a single nuisance regressor for the entire brain (such as global signal regression) due to the clear regional heterogeneity of the physiologically-coupled responses. These results challenge previous notions that physiological confounds are either localized to large veins or globally coherent across the cortex, therefore emphasizing the necessity to consider potential physiological contributions in fMRI-based functional connectivity studies.
Perception and Language

Xiangbin Teng
Constrained Structure of Ancient Chinese Poetry Facilitates Speech Content Grouping
Ancient Chinese poetry is constituted by structured language that deviates from ordinary language usage; its poetic genres impose unique combinatory constraints on linguistic elements. How does the constrained poetic structure facilitate speech segmentation when common linguistic and statistical cues are unreliable to listeners in poems? We generated artificial Jueju, which arguably has the most constrained structure in ancient Chinese poetry, and presented each poem twice as an isochronous sequence of syllables to native Mandarin speakers while conducting magnetoencephalography (MEG) recording. We found that listeners deployed their prior knowledge of Jueju to build the line structure and to establish the conceptual flow of Jueju. Unprecedentedly, we found a phase precession phenomenon indicating predictive processes of speech segmentation—the neural phase advanced faster after listeners acquired knowledge of incoming speech. The statistical co-occurrence of monosyllabic words in Jueju negatively correlated with speech segmentation, which provides an alternative perspective on how statistical cues facilitate speech segmentation. Our findings suggest that constrained poetic structures serve as a temporal map for listeners to group speech contents and to predict incoming speech signals. Listeners can parse speech streams by using not only grammatical and statistical cues but also their prior knowledge of the form of language.
Yuanning Li
The encoding of linguistic pitch patterns in human superior temporal gyrus
In tone languages such as Mandarin Chinese, the pitch trajectory of a syllable distinguishes word meanings. The neural computations underlying lexical tone representation in the human auditory speech cortex are unknown. To address this, we used high-density electrode arrays to examine cortical activity in native Mandarin-speaking participants while they listened to natural, continuous Mandarin speech. We found that neural activity at single electrodes over the superior temporal gyrus (STG) did not represent tone categories, but rather high-order auditory processing of speaker-normalized pitch (height and change). Similar encoding of pitch was observed when the same participants listened to natural English speech. At the population-level of STG responses, however, we found neural sensitivity to tone categories. This was more prominent in native Mandarin-speakers compared to a control group of English-speakers who listened to the same stimuli. Furthermore, cortical responses in Mandarin-speakers encoded a longer temporal integration window for pitch. These cross-linguistic results demonstrate that the neural representation of lexical tones relies upon general language-independent auditory processing at local sites, but as a collective ensemble, form an emergent language-specific representation to support speech perception.
Nihong Chen
Flexible perception: functional plasticity in the human brain
Training and experience improve our perceptual skills. Yet the functional brain architecture that supports this process is little understood. Using psychophysics, fMRI, TMS, and intracranial recordings, our work demonstrates extensive cortical plasticity in human adults: 1) learning refines the sensory representation in the visual cortex; 2) learning enhances the cortico-cortical communication between the visual cortex and the high-level decision-making related area; 3) learning modifies the inherent functional specializations of visual cortical areas; 4) exposure induces a cue-triggered replay in the early visual cortex; 5) learning ameliorates the crowding effect in peripheral vision. These findings suggest that perceptual experience shapes the functional architecture of the brain in a much more pronounced way than previously believed.
Pinglei Bao
A unified model for understanding the functional organization of inferotemporal cortex
How is the representation of complex visual objects organized in inferotemporal (IT) cortex, the brain region responsible for object recognition? Areas selective for a few categories such as faces, bodies, and scenes have been found, but large parts of IT lack any known specialization, leading to uncertainty over whether any general principle governs IT organization. Here, we used fMRI, microstimulation, electrophysiology, and deep networks to investigate the organization of macaque IT. We built a low dimensional object space to describe general objects using a deep network. Responses of IT cells to a large set of objects revealed that single IT cells are projecting incoming objects onto specific axes of this space. Remarkably, cells were anatomically clustered into four networks according to the first two components of their preferred axes, forming a map of object space. This map was repeated across three hierarchical stages of increasing view invariance, and cells comprising these maps collectively harbored sufficient coding capacity to reconstruct arbitrary objects. These results provide a unified picture of IT organization in which category-selective regions are part of a coarse map of object space.
Social Neuroscience
Lihui Wang
Explorative action representations in high-level visual cortex
A traditional approach to visual perception is to map the physical attributes onto the visual cortex, whereas the concurrent action of the agent during visual exploration is assumed to play little role in the visual cortex. Here we show that eye movement - i.e. motor - sequences elicited while looking at faces or houses elicit distinct activation patterns in visual perceptual areas in ventral occipitotemporal cortex. In a series of experiments, eye-movements were firstly recorded while observers were looking at faces or houses. These eye-movement tracks were then replayed in the form of a dot moving on a uniform background that observers had to follow with their gaze. The brain activity in the fusiform face area (FFA) and the parahippocampal place area (PPA) showed distinct patterns to face- and house-related gaze-tracks that could be discriminated with multivariate pattern analysis (MVPA), in the absence of any face or house images. Moreover, the discrimination worked best when observers retraced their own eye-movement patterns, compared to patterns elicited by other observers. These findings suggest that the high-level visual cortex represents the action sequences that are used to explore the visual categories.
Jiefeng Jiang
Prefrontal reinstatement of contextual task demand is predicted by separable hippocampal patterns
Goal-directed behavior requires the representation of a task-set that defines the task-relevance of stimuli and guides stimulus-action mappings. Past experience provides one source of knowledge about likely task demands in the present, with learning enabling future predictions about anticipated demands. We examine whether spatial contexts serve to cue retrieval of associated task demands (e.g., context A and B probabilistically cue retrieval of task demands X and Y, respectively), and the role of the hippocampus and dorsolateral prefrontal cortex (dlPFC) in mediating such retrieval. Using 3D virtual environments, we induce context–task demand probabilistic associations and find that learned associations affect goal-directed behavior. Concurrent fMRI data reveal that, upon entering a context, differences between hippocampal representations of contexts (i.e., neural pattern separability) predict proactive retrieval of the probabilistically dominant associated task demand, which is reinstated in dlPFC. These findings reveal how hippocampal-prefrontal interactions support memory-guided cognitive control and adaptive behavior.
Lei Zhang
Neurocomputational mechanisms of social influence in goal-directed learning
Humans learn from their own trial-and-error experience and from their social partners to acquire reward values. However, direct learning and social learning were often studied in isolation, and it remains unanswered how brain circuits compute and integrate expected values when direct learning and social learning coexist in an uncertain environment. Here, I will present a real-time multi-player goal-directed learning paradigm, where, within each group five, one participant was scanned with MRI (overall n = 185, MRI n = 39). We first observed opposite effects of group consensus on choice and confidence. Leveraging reinforcement learning and fMRI we captured nuanced distinction between direct valuation through experience and vicarious valuation through observation, and their dissociable, but interacting neural representations in the vmPFC and the ACC, respectively. Connectivity analyses revealed increased functional coupling between the right temporoparietal junction (rTPJ) representing instantaneous social information and the putamen, when individuals made behavioral adjustment as opposed to when they stuck with their initial choice. We further identified that activity in the putamen instantiated a hitherto uncharacterized social prediction error, rather than a reward prediction error. These findings suggest that an integrated network involving the brain’s reward hub and social hub supports social influence in goal-directed learning.
Yingying Han
How do the emotions of others affect us?
The human anterior cingulate cortex (ACC) responds while experiencing pain in the self and witnessing pain in others, but the underlying cellular mechanisms remain poorly understood. Here we show the rat ACC (area 24) contains neurons responding when a rat experiences pain as triggered by a laser and while witnessing another rat receive footshocks. Most of these neurons do not respond to a fear-conditioned sound (CS). Deactivating this region reduces freezing while witnessing footshocks to others but not while hearing the CS. A decoder trained on spike counts while witnessing footshocks to another rat can decode stimulus intensity both while witnessing pain in another and while experiencing the pain first-hand. Mirror-like neurons thus exist in the ACC that encode the pain of others in a code shared with first-hand pain experience. A smaller population of neurons responded to witnessing footshocks to others and while hearing the CS but not while experiencing laser-triggered pain. These differential responses suggest that the ACC may contain channels that map the distress of another animal onto a mosaic of pain- and fear-sensitive channels in the observer. More experiments are necessary to determine whether painfulness and fearfulness in particular or differences in arousal or salience are responsible for these differential responses.
Computational Cognitive Science

Yunzhe Liu
Neural Replay in Abstraction and Inference
Humans exhibit remarkably flexible behaviour. We can choose how to act based on experiences that are only loosely related and imagine the consequences of entirely novel choices. Such flexibility is thought possible because the brain builds internal models of the world (i.e., cognitive map) that accounts for the relationships between isolated experiences that enable a generalization of knowledge to new situations. How the brain builds and updates a world model remains a central question in cognitive neuroscience. Replay and/or Preplay is one proposed mechanism. In the replay, patterns of cellular firing during rest spontaneously play out past spatial trajectories in both forward and reverse directions, and reverse replays are increased for rewarded trajectories. In preplay, potential future trajectories are played out, perhaps constrained by structural knowledge of relationships in the world. This computation is also implied by Dyna-type learning algorithms. However, despite the theoretical importance of neural replay/preplay, its study has so far been largely restricted to spatial navigation tasks in rodents. It is unknown whether knowledge of task structure can indeed impact on the sequences that are (pre- or re-) played. In this talk, I will show two studies conducted in humans using magnetoencephalography (MEG) to measure fast spontaneous sequences of representations. By building pattern classifiers of MEG sensor activity for each stimulus, we detected sequential reactivation of their trajectories during rest. These sequences recapitulated known features of neural replay and reflected correctly re-assembled orderings, rather than experienced trajectories, this supports the notion that task structural knowledge imposed a constraint on replay. We provide further evidence that neural pre-play is a manifestation of abstract structure knowledge, and the representation in the replay is factorised so that a sensory code of object representations was preceded 50 ms by a code factorized into sequence position and sequence identity. The forward replay of a correctly re-assembled sequence transitioned to that of reverse replay when a sequence was rewarded. Together they help build, maintain and update the world model. If time permits, I will also show some replay data in episodic memory retrieval, and its implications in psychiatric disorder, like Schizophrenia.
Dalin Guo
Features or Bugs? Synergistic Idiosyncrasies in Human Learning and Decision-Making
Humans and animals frequently need to make repeated choices among imperfectly known options, thus negotiating a tension between exploration and exploitation. Based on self-reported reward estimation and computational modeling of choice behavior in a bandit task, we find that human subjects systematically underestimate true reward rates, especially when the reward is abundant. Additional analyses reveal that this underestimation compensates for two other apparent suboptimalities: a default assumption of environmental non-stationarity, and the adoption of a simplistic decision policy. The combination of these three idiosyncrasies allows humans to achieve near-optimal performance while reaping the benefits of computational efficiency and behavioral flexibility. Furthermore, we show that the human tendency to overestimate environmental nonstationarity, captured by the best-fitting Bayesian hidden Markov model, is equivalent to a particular class of reinforcement learning algorithms, variants of which researchers have found to be good empirical fits to data. Broadly speaking, this work makes two significant contributions to neuroscience: (1) the discovery and an explanation of how the brain synergistically combines multiple suboptimalities to compensate for one another and achieve near-optimal behavior, (2) a theoretical framework that yields statistically grounded and unified interpretations of human and animal behavior that have been fit using reinforcement learning algorithms.
Computational Neuroscience
Wenhao Zhang
A normative theory of causal inference in multisensory integration in neural circuits
Causal inference is important in cognition and perception. In perceptual inference such as cue integration, the nervous system needs to infer the underlying causal structures of whether the cues are from the same or different sources, and based on which to choose whether to integrate or segregate inputs from different sensory modalities. It is still an open question in computational neuroscience that how neural circuits implement the causal inference. To shed light to this question, we consider the causal inference in multisensory processing and propose a novel generative model based on neural population code which takes into account both stimulus feature and stimulus strength in the inference. In the case of circular variables such as heading direction, our normative theory yields an analytical solution with a clear geometric interpretation, and can be implemented by simple additive mechanisms in neural population code. Numerical simulation shows that the tunings of the neurons inferring the causal structure are consistent with the "opposite neurons" discovered in dorsal medial superior temporal (MSTd) and the ventral intraparietal (VIP) areas for visual-vestibular processing. This study illuminates a potential neural mechanism for causal inference in the brain.
Wenliang Li
Take a moment: representation of perceptual uncertainty in dynamic inference
The brain is capable of extracting relevant information from noisy and ambiguous signals and produces percepts. Although this process shows features of optimal inference, how the brain represents and computes with uncertainty is largely unknown. Here we develop a moment representation of uncertainty that supports accurate and flexible representation of beliefs in dynamic situations, and can implement a class of computation known as postdiction, a natural mode of perceptual inference by the brain.
Chengcheng Huang
Circuit models of low dimensional shared variability in cortical networks
Neuronal variability is a reflection of recurrent circuitry and cellular physiology, and its modulation is a reliable signature of cognitive and processing state. A pervasive yet puzzling feature of cortical circuits is that despite their complex wiring, population-wide shared spiking variability is low dimensional with all neurons fluctuating en masse. Previous model cortical networks are at loss to explain this variability, and rather produce either uncorrelated activity, high dimensional correlations, or pathologically network behavior. We show that if the spatial and temporal scales of inhibitory coupling match known physiology then model spiking neurons naturally generate low dimensional shared variability that captures in vivo population recordings along the visual pathway. Further, top-down modulation of inhibitory neurons provides a parsimonious mechanism for how attention modulates population-wide variability both within and between neuronal areas, in agreement with our experimental results. Our theory provides a critical and previously missing mechanistic link between cortical circuit structure and realistic population-wide shared neuronal variability.
Guangyu (Robert) Yang
Machine Evolving Olfactory Systems
Flies and mice are separated by 600 million years of evolution yet they have evolved olfactory systems that share many anatomic and functional features. The similarity of the olfactory systems in evolutionarily distant organisms may reflect a common drive to enable rapid learning of novel olfactory associations and to elicit innate behavioral responses to salient odors. We asked whether networks constructed by the machine learning to perform olfactory tasks converge on the same structural organization as these natural olfactory systems. Artificial network trained to classify odor identity recapitulate structural principles inherent in the olfactory system, including input units driven by a single receptor type, the convergence of similarly responding input units onto 'glomeruli', and sparse unstructured connectivity to a large third-layer representation.